Feedback about the challenge
Pros:
-The referee code was super useful. This is a must have from now on. There are so many little things that the only way to have it right is by having it.
-Fast matches, 1v1 symmetric that are the most fair.
-50ms is good for fast matches…
-2 full weeks are nice for many people.
Cons:
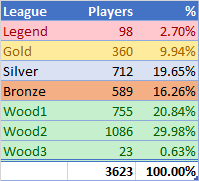
-Wood leagues have more than 50% of players.
I think this is frustrating for these players. The real challenge should start at bronze and not wood. I now there was the twitch video, but is not heavily promoted. So to avoid player frustation, reaching bronze should be easier.
-Crazy hard legend bot, only 13 players passed at first batch, so it was like a legend 15th bot. This makes a lot of good players can’t pass from gold, when they deserve it. This was another BIG point of frustation, for me it’s a no-no.
-Ended on monday at like 3-5am in America, it’s a bad time.
-Bad timeouts at sunday? Suddenly my bot started losing a lot due to timeouts, I changed nothing.
EDIT: -Being 335th/3624 on this challenge gives more points that being 1st/1669 on a low played challenge like Codebusters. Now tell me that being 1st on CB is easier than 335th on Pirates. This is a BIG problem for correct rankings. Scoring isn’t fair for low played challenges.
About my bot
Final rank 40th legend.
I worked on this bot from monday to sunday, about 60hrs of work and 2200+ lines of code (I’m a bad, slow coder).
Most of the time was for polishing the simulator. Even with the referee code, converting it to another language isn’t trivial. Java manages List of objects as references, whereas a C++ vector is a hard copy. This makes me waste 1 day on searching why collisions got on an endless loop. Another thing was the difference between double values on both languages. The function angle() gives different results on C++, that leads to a different moveTo() outcome.
I precalculated a lot of things on first turn, mainly distance() and angle(), that are the most expensive. Both were a 2D array, like dist[pointA][pointB]. I coded coords to index with (((y+2)<<5)+x), the +2 was to avoid out of bounds on the array due to negative positions on bow() or stern() I have a bigger array than the map due to the index wasting space with the <<5. I also coded neighbours on an array, neigh[point][orientation].
Once I had a simulator I started testing it with my own bot as enemy. As I know both movements, I can apply them to both simulations and the resulting GameState update must match with the next turn on the game. I had problem with that due to mines, because they aren’t seen at more than dist = 5 (another day wasted).
All this took me +40hrs unfortunately, so I needed to rush the AI.
My AI is an almost pure Genetic Algorithm (GA) for move actions, WAIT and MINE with this genoma:
class Genoma {
//Only myShipCount used for GA, not always MAX_SHIPS. MAX_DEPTH was 5
Action action[MAX_SHIPS][MAX_DEPTH];
, with FIRE being used manually when I have a WAIT as an action for one ship. Monte Carlo also worked fine, but on my final submit it was a GA.
WAIT is like free time to do shootings, so I manually wrote some basic targeting heuristics to shoot at future position of the enemy, I first tried to replicate the moveTo actions for enemies, guessing they’ll target the nearest rum barrel. But it didn’t work very well with speed=2 ships, so in that case I try to shoot a barrels near the enemy and farther from my ships. A late addition was the manual sacrifice of ships, ships drop rum when killed (up to 30) so I checked if I was losing (less health than the enemy), in that case the ship with the least rum shoot itself, and mark its id as sacrificied. This way I don’t do double shooting or anything.
About the fitness function, I have two stages, with or without barrels. With barrels I priorize speed, health and rum captures, and penalize distance to nearest rum barrel. That makes ships goes naturally to the nearest barrel. I also gives bonus to my ship with the most rum. For the mine placement I didn’t have time to do something good so I just penalize a lot if the ship has mineCooldown, that makes ships only place mine if they think it will hit an enemy. For enemy ships I penalize this health.
Once there are no barrels I keep all the above but added more scores. I go to cat & mouse game, If I’m winning OR the total health of my ships are greater than enemy’s total health, I run away. I score positively speed, distance between enemy ships and my ships, and penalize distance between my ships, That makes my ships tries to stay near, but away from the enemy. Then I have the right conditions to sacrifice the ship with least life, and buff the bigger.
If I’m losing, then I penalize distance between enemy ships and mine, just that.
I have many flaws, specially with FIRE, I target so badly that I even hit myself. I don’t focus on fire a single enemy too.
I don’t trap ships, bad mines, etc etc.
But I’m happy with the results, the GA made some nice moves. My whole AI doesn’t have a real pathfinding because map is simple, so it’s done by the GA. And that means they can run into a mine, or take hits if with that the AI thinks it’s a better move.
If you see from frame 142 in that replay https://www.codingame.com/replay/212958220 you’ll see how one of my ships just go for one mine to die. That’s not intended by me or hardcoded, but the GA thinks it’s better to “sacrifice” a ship and buff the biggest ship. The other ship at turn 156 did the same, but this was enough to win the game.
And my ships also got hit on purpose to die (again without a manual sacrifice), like on this https://www.codingame.com/replay/212958005 turns 172/174.
GA (or even Monte Carlo) can do nice stuff, stupid moves that ends well. This is hardly done with pure heuristics, because it’s not easy to do. But you need an almost perfect simulator, or things can go terribly wrong.
I don’t simulate offline at all, neither do fine tuning of coefficients. All was done on Notepad++, and testing only on CG IDE and sometimes CG spunk.
I recommend new players they learn about Monte Carlo and Genetic Algorithms, they are really interesting search algorithms. Codingame is about to release a new learning platform, and I’m pretty sure there will be a lot of nice courses about these subjects. E.g. a fast hands-on for Monte Carlo I wrote: https://tech.io/playgrounds/4003e618997c561e705af1ade9cfca4b432/monte-carlo-method-in-7-minutes (beta version, can change in the future). Stay tuned 